Why It is Important to Understand Different Machine Learning Algorithms in 2025?
The growing influence of technology and the overreliance on AI solutions in various industries have made it crucial to understand different machine learning algorithms. In 2025, AI-assisted innovations will transform the world, making choosing the right algorithms for the right tasks more essential than ever.
Whether you’re looking to enhance automation, optimize decision-making, or improve predictive analytics, selecting the appropriate and relevant machine learning approach can substantially impact efficiency and accuracy.
Moreover, machine learning is a vast field, offering several types and algorithms, each designed to serve a unique purpose. We know how important it is to have a solid grasp of these techniques to build more secure, quicker, and reliable solutions. In this article, we will talk about different machine learning algorithms and why mastering them is crucial in 2025.
Table of Contents
What are Machine Learning Algorithms?
Machine learning algorithms are computational methods that help the computer to learn patterns from a vast database. Moreover, it also enables computers to make decisions and predictions without any programming. In addition to that, these algorithms interpret input data, identify patterns, and optimize models over time to enhance accuracy.
Generally, these come under different categories such as supervised learning, unsupervised learning, semi-supervised learning, and reinforcement learning.
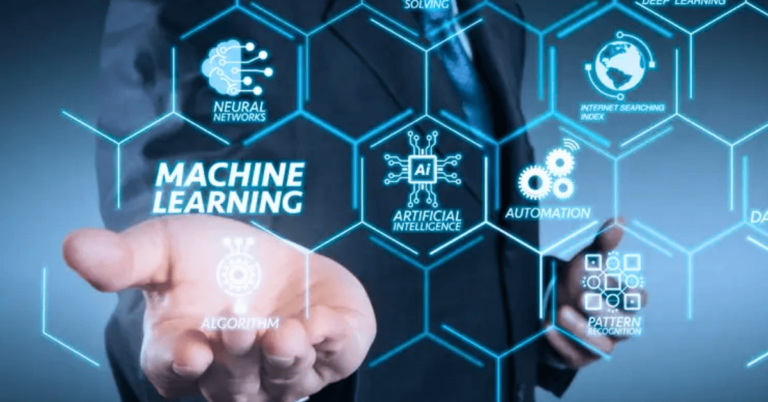
For example:
Let’s understand with an example. In supervised learning, a spam detection algorithm is run to classify emails as spam or not. Moreover, in unsupervised learning customer segmentation, algorithms are used to group users with the same purchasing behavior without any labeled data.
How Algorithms are the Backbone of AI Systems?
Algorithms are the backbone of AI systems because these are the building blocks of AI solutions. Such as:
- These algorithms allow AI systems to analyze data, interpret patterns, and make informed decisions.
- They are involved in automating repetitive and time-consuming tasks, improving efficiency and accuracy.
- Machine learning algorithms continuously learn from data to improve performance over time.
- Additionally, these algorithms allow AI systems to process large datasets efficiently
The Role of Machine Learning in the Current Era
We know that machine learning has become a driving force for various technological advancements. Moreover, it is very much involved in our daily lives. For instance, it is present in our phones to assist us, and in cars to help us navigate and automate our routine tasks.
Moreover, it has revolutionized almost every industry. In healthcare, it offers AI-driven diagnosis, personalized treatment, and predictive analytics. Moreover, in finance, it helps us in fraud detection, risk assessment, and trading. Furthermore, smart robotics and AI-powered decision-making have changed the automation industry.
Additionally, with the rise of big data, deep learning, and AI-powered decision-making, mastering machine learning is more crucial than ever. Several emerging trends such as explainable AI, federated learning, and edge AI emphasize the growing demand for algorithm knowledge.
Why it is Important to Understand Different Machine Learning Algorithms?
It is important to understand different machine learning algorithms because it helps individuals and organizations learn how they work for specific tasks, their applications, their limitations, and how to use them efficiently.
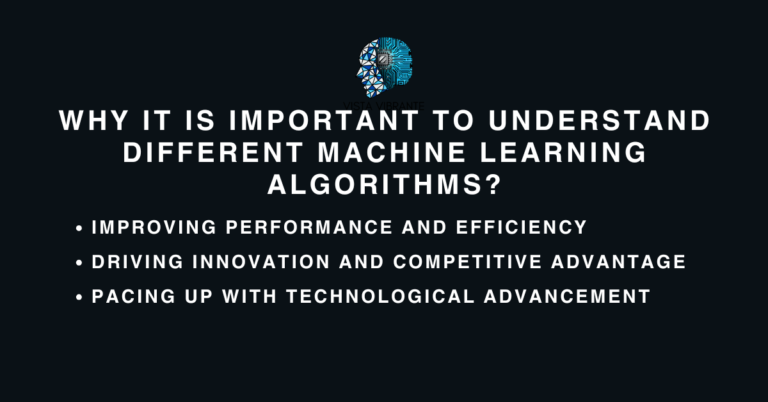
-
Choosing the Correct ML Algorithm for the Right Problem:
Different algorithms are designed to cater to different machine learning tasks such as classification, and regression or clustering. It ensures accuracy, efficiency, and relevance in solving real-world problems.
For example: In predicting land value, the regression model of supervised learning will be used as it will forecast the price fluctuations and other related factors.
-
Improving Performance and Efficiency
Identifying and understanding different algorithms would help to improve overall performance and solve problems efficiently. Choosing the right one will yield the best results without any additional errors.
For instance, poor algorithm selection can result in inaccuracies, biases, and inefficiency.
-
Driving Innovation and Competitive Advantage
Machine learning drives innovation and helps individuals to make groundbreaking AI solutions. Companies using the right algorithms acquire a competitive advantage in automation, personalization, and decision-making.
-
Pacing up with Technological Advancement
AI and machine learning are continuously evolving which gives rise to new algorithms to handle complex data and tasks. Staying updated with these algorithmic advancements helps you remain relevant and adaptable in the ever-evolving AI space.
Types of Machine Learning Algorithms
Machine learning algorithms are subsets of AI that deal with different algorithms to make an efficient solution. These algorithms are classified majorly into supervised, unsupervised, reinforcement, and Deep learning.
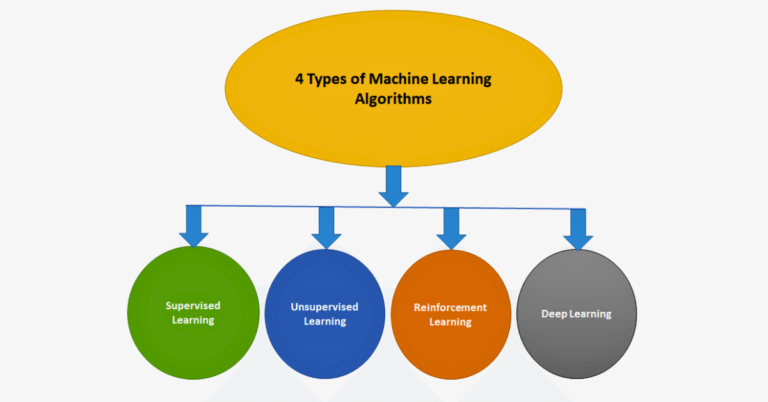
1. Supervised Learning Algorithms
Supervised Learning algorithms usually work on the labeled data. The model is trained on predefined data to give us accurate output. In short, in this, an algorithm learns to map inputs to output according to given training.
- These algorithms are used for classification and regression tasks.
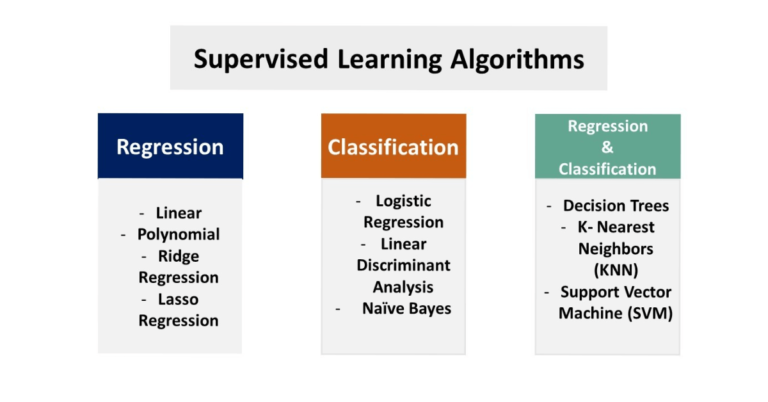
Here are some common supervised learning algorithms:
1. Linear Regression
- A regression algorithm is used to predict the relationship between input features and output by fitting a linear line to minimize prediction errors.
- Such as estimating house prices based on square foot, number of bedrooms, etc
2. Logistic Regression
- It is a classification algorithm that is used to classify data into binary categories using a logistic function, such as “0 & 1“
- It is used for binary classification tasks such as spam detection, suggesting emails as spam or not.
3. Decision Tree
- It works by creating a tree-like model to make decisions based on input features that are easy to interpret.
- For example, we used this algorithm in customer customer segmentation based on age, income, or buying behavior.
4. Support Vector Machines (SVM)
- It is regarded as the most powerful algorithm that classifies data by finding the hyperplane that separates different classes.
- It is used in face recognition, text classification tasks
5. Random Forest
- It is an ensemble learning method that combines different decision trees to improve accuracy and avoid overfitting.
- For instance, it is used in fraud detection and customer segmentation.
6. K-Nearest Neighbors
- Well, it is the most simple, non-parametric algorithm that separates a data point according to the majority class of its nearest neighbors in the feature space.
- For example, product recommendation systems, where the same items are recommended based on user preferences.
7. Naive Bayes
- This algorithm is used when you’re assuming a feature independence or doing predictive modeling.
- It is a probabilistic classifier based on Bayes theorem to make text classification efficient and easy.
- For instance, its popular use case is carrying out sentiment analysis in social media.
8. Gradient Boosting Machines (GBM)
- GBM also used an ensemble technique to build a tree sequence and correct errors from the previous version for great accuracy.
- It is used to detect fraud in financial transactions.
9. XGBoost (Extreme Gradient Boosting)
- It is the advanced and optimized version of the gradient boosting aid to bring speed and performance to extensive datasets.
- An example of this algorithm is click-through rate prediction in digital marketing.
10. Artificial Neural Networks (ANNs)
- Deep-learning models are composed of neuron layers designed to handle complex patterns in high-dimensional marketing.
- The most common example is speech recognition in virtual assistants like Siri and Alexa.
Real-World Application of Supervised Learning Algorithms
Here are some real-world examples of supervised learning algorithms:
Industries |
Key Application |
Healthcare |
|
Finance |
|
Retail & Commerce |
|
Marketing & Advertising |
|
Security and Surveillance |
|
2. Unsupervised Learning Algorithms
This algorithm model works on unlabeled data. The model is trained on data to find hidden patterns and relationships. An unsupervised learning algorithms model is tasked to process raw data with input without any output parameters.
- It analyzes and predicts based on its knowledge
- Uncovers facts that can be hidden and covered in the labeled data sets.
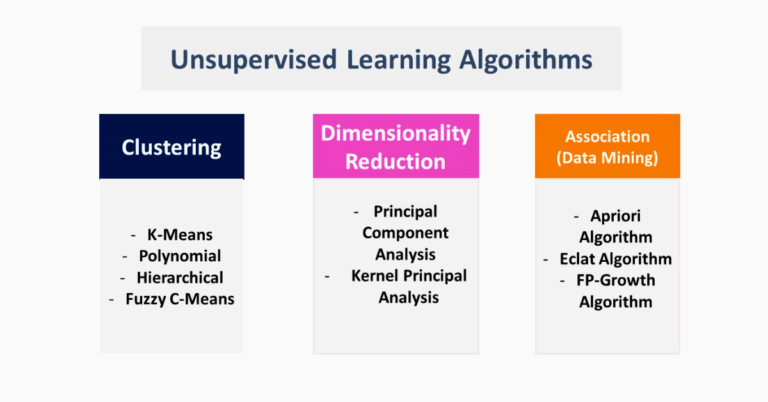
Let’s explore some popular unsupervised machine-learning algorithms:
1. K-Means Clustering
- It is a type of clustering that uses partition-based clustering modes to assign data points into K clusters based on feature similarity.
- For example, it is used in customer segmentation to group shoppers with the same purchasing habits.
2. Hierarchical Clustering
- In this type, a hierarchy of clusters is built using a top-down or bottom-up approach without mentioning the number of clusters beforehand.
- For example, this algorithm is used to classify genes with similar expression patterns.
3. Principle Component Analysis (PCA)
- It is a dimensionality reduction technique that converts high-dimensional data into fewer principle components while preserving its characteristics.
- A key example is it is used in facial recognition to reduce image features while preserving its key elements.
4. Autoencoders
- It is a type of neural network that is used to compress input data into less dimensional form and then reconstruct it.
- For example, this algorithm has common usage in anomaly detection or finding any unusual pattern in transactions.
5. Gaussian Mixture Model
- It is a type of probabilistic clustering that predicts data generated from various Gaussian distributions, allowing it to be used for soft clustering.
- It is applied in speech recognition to differentiate between different voices.
6. Apriori Algorithm
- It is an association rule learning technique that discovers emerging patterns by identifying constantly occurring itemsets in large datasets.
- It is applied for market basket analysis to identify relationships between purchased items.
Real-world Applications of Unsupervised Learning Algorithms
Here are some real-world examples of Unsupervised Learning Algorithms:
Industries |
Key Application |
E-commerce & Marketing |
|
Healthcare & Genomics |
|
Finance & Banking |
|
Cybersecurity |
|
Streaming & Entertainment |
|
3. Reinforcement Learning (RL) Algorithms
RL is the machine algorithm that uses a trial-and-error method. It does not rely on input and output data. The machine directly interacts with the physical environment and decides based on its learning process.
At the end of the result, feedback is provided to the computer so it adjusts its decision or results based on the provided feedback.
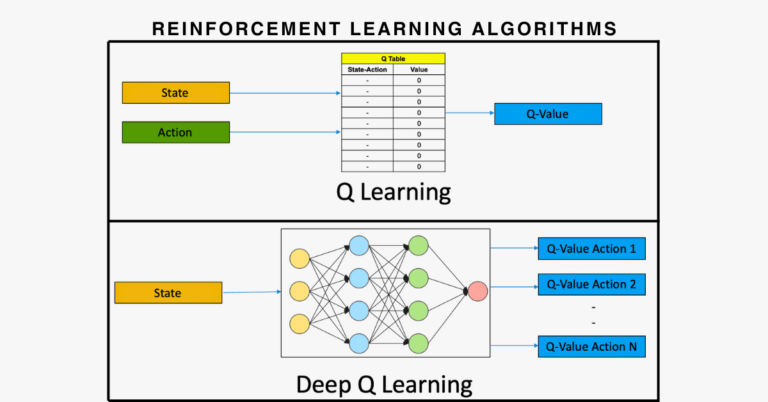
Some common Reinforcement Learning algorithms are given below:
1. Q Learning
- It is a value-based RL algorithm that learns from an optimal-action selection policy using a Q-table.
- It is commonly used in game AI and robotics control.
2. Deep Q-Networks (DQN)
- It is a popular algorithm that is an extension of Q-learning used to deploy neural networks to manage high-dimensional state spaces.
- Well, it is commonly applied in autonomous driving and static game playing.
4. Deep Learning Algorithms
It is a subset of machine learning algorithms that works on neural networks. This field is inspired by the neuroscience – field of biology, which deeply digs into layers and performs classification, regression, and deep learning tasks.
It uses artificial neural networks and models to represent the data in hierarchical patterns.
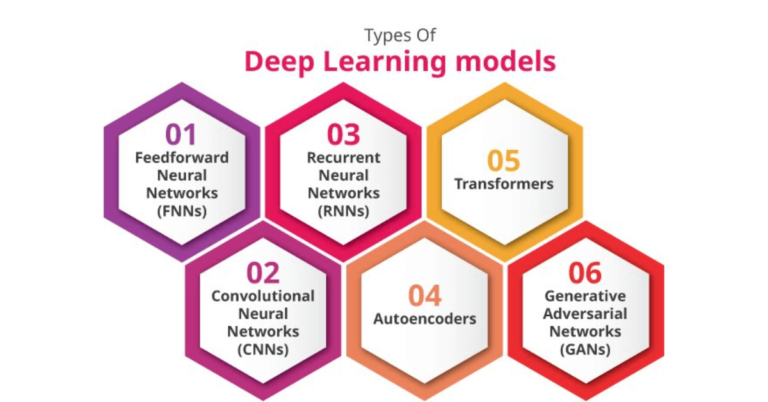
Some common types of Deep Learning Algorithms include:
1. Transformer-Based Models
- A transformer network is a deep learning algorithm that is used for processing sequential data and they rely only on self-attention machines and positional encoding.
- Particularly, it is used for natural language processing (NLP) tasks such as Google translations or text generation from GPT.
2. Convolutional Neural Networks (CNNs)
- It is used for specialized image and spatial data processing by using convolutional layers to detect patterns like edges and textures.
- It is commonly used for computer vision tasks like image recognition, and medical imaging.
3. Recurrent Neural Networks (RNNs)
- It is used when you need to retain past information in sequential data through loops, making them valuable in time-series tasks.
- It is used in speech recognition for virtual assistants like Siri.
4. Generative Adversarial Networks (GANs)
- At current times, it is a widely used algorithm that consists of a generator and discriminator to create realistic synthetic data when working together.
- For instance, AI-generated content from ChatGPT or AI-generated artwork.
What are the Factors Behind Selecting a Machine Learning Algorithm?
There is always a question about which algorithm best suits a particular problem. To identify and classify the problem and choose the correct algorithm, consider these factors.
-
Nature of the Data
The nature of data should be analyzed by checking the sample sizes of data sets and their distribution and dimensions.
-
Desired Outcomes
We need to understand what is required to generate the result. An algorithm is chosen per desired outcomes requirements as a decision tree is required to portray the relationship or occurrence chances of an event through probability.
-
Computational Requirements and Time
Some algorithms take time to run or require more data and power, so they need to be identified according to the time and computational requirements.
How can we Stay Updated with Machine Learning Algorithms in 2025?
In 2025, staying updated with machine learning algorithms is very crucial because it helps you drive innovations and come out with amazing solutions.
-
Exploring Online Resources:
Self-study is the best way to keep yourself up-to-date with the latest trends. You can also follow research papers and publications to learn about new research and advancements in machine learning.
For example, you stay informed through journals like IEEE, and NeurIPS and read blogs and articles from AI research labs such as Google AI, OpenAI, and DeepMind.
-
Participate in Online Courses and Certifications
You can also opt for different ML and AI certifications on platforms like Coursera, Udacity, and edX.
Moreover, you can also enroll in specialized courses on Reinforcement Learning, Deep Learning, and NLP.
-
Practice ML Frameworks & Tools
Practice is the key to success. You can practice with tools like TensorFlow, PyTorch, and Scikit-learn for applying machine learning skills and concepts to real-world datasets and open-source projects.
-
Attend Conferences and Webinars
Participating in live events, seminars, and industry conferences, such as CVPR, ICML, and AAI. It will help you gain knowledge of trends and the latest practices taking place in the AI landscape.
Conclusion
In 2025, machine learning is not a buzzword, but a reality that is driving innovation across industries with its global adoption projected to grow by 35% annually. Moreover, a Gartner report stated that 80% of enterprises are deploying AI and ML-driven solutions. This shows the dire need for a deep understanding of machine learning algorithms.
Our team has gone through every single aspect of this topic and gathered all relevant information to craft this article. Whether you want to learn about supervised machine learning algorithms, unsupervised, or more, this article is perfect for building a quick understanding.
FAQs
What is the simplest Machine learning Algorithm?
The most straightforward machine algorithm is linear regression, which is a type of supervised learning algorithm. It identifies the relationship between variables, one dependent and the other being one or more than one considered an independent variable.t.
What are the categories of machine learning algorithms?
Generally, there are three main categories of machine learning algorithms referred to as supervised, unsupervised, reinforcement learning, and deep learning algorithms.