The Best Applications of AI in Finance for Enhanced Decision-Making
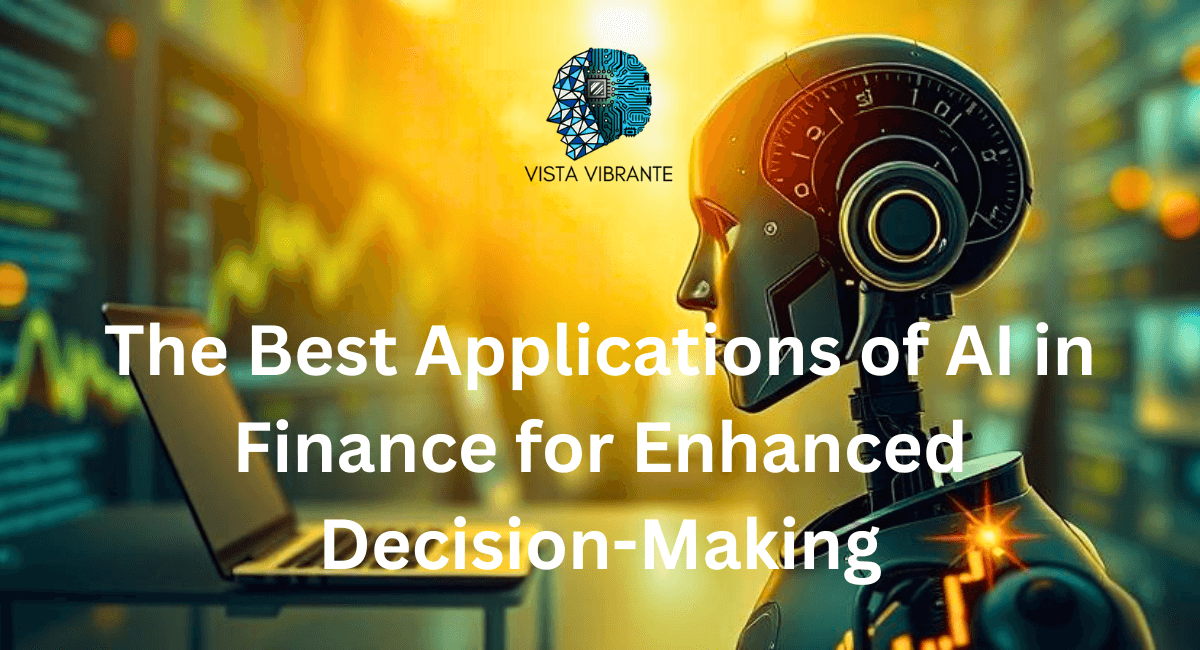
AI is changing the financial services industry, making decisions, and improving the customer experience. AI-powered solutions allow financial institutions to process vast amounts of data, perform analytics, assess risks, detect fraud, and personalize.
AI in finance is used for performance measurement, predictive modeling, and financial forecasting. It helps organizations predict market trends and optimize investment strategies. AI also improves operational efficiency by automating routine tasks, reducing errors and improving regulatory compliance.
Also, powered chatbots and virtual assistants improve customer interaction by providing instant support and financial advice. Machine learning algorithms analyze customer behavior, enabling banks and financial institutions to offer personalized solutions.
AI in Finance Timeline
- 80s-90s: Credit scoring and fraud detection
- 2000s: Algorithmic trading
- 2010s: Chatbots, robo-advisors, and risk assessment
- 2020s- Now: Generative AI, blockchain, and regulatory compliance
AI is coming and will make better decisions that are more secure and efficient.
Machine Learning in Finance
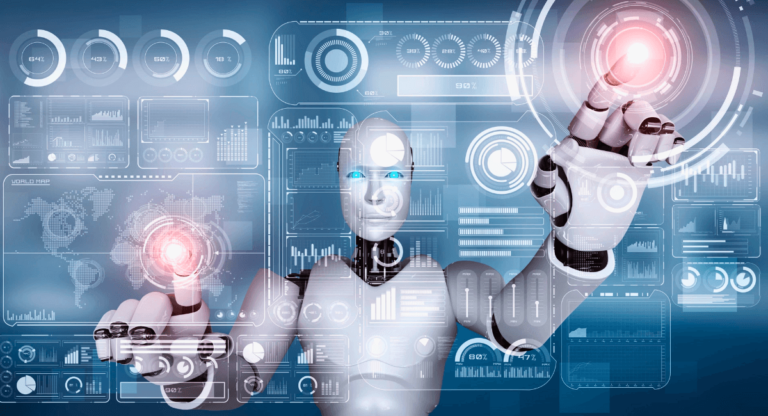
ML is a branch of AI that lets financial institutions process big data, find patterns, and make decisions. ML is getting more effective in finance with neural networks and deep learning.
Financial institutions use ML algorithms for risk, fraud, credit scoring, and algorithmic trading. These models find anomalies in transactions, detect fraud, and enhance security. ML automation also automates, reduces errors, and increases speed.
ML also personalizes financial products and services based on user behavior. Banks and investment firms use it to optimize portfolio management and predict market movements.
Financial institutions can refine their strategy and make better decisions by training models with historical and real-time data. As ML improves, its role in finance will only get bigger and better, reshaping the industry with more innovative data-driven solutions.
Applications of AI in the Finance Industry
Artificial Intelligence (AI) is changing the financial landscape by making things more efficient, cost savings, and better customer experiences. AI technologies allow financial institutions to personalize services, create new opportunities, and manage risk.
One of the main applications of AI in finance is fraud detection, where machine learning algorithms analyze transactions in real time to detect anomalies and prevent fraud. AI also helps with risk management by predicting potential financial threats and assisting organizations to make data-driven decisions.
AI also automates, reduces manual tasks, and streamlines processes like loan approvals, credit scoring, and regulatory compliance. It improves customer service with AI-powered chatbots and virtual assistants, personalizing financial advice and providing instant support.
By being transparent and compliant with regulations, AI builds trust and accountability in financial services. As AI evolves, its applications in finance will grow and shape the industry.
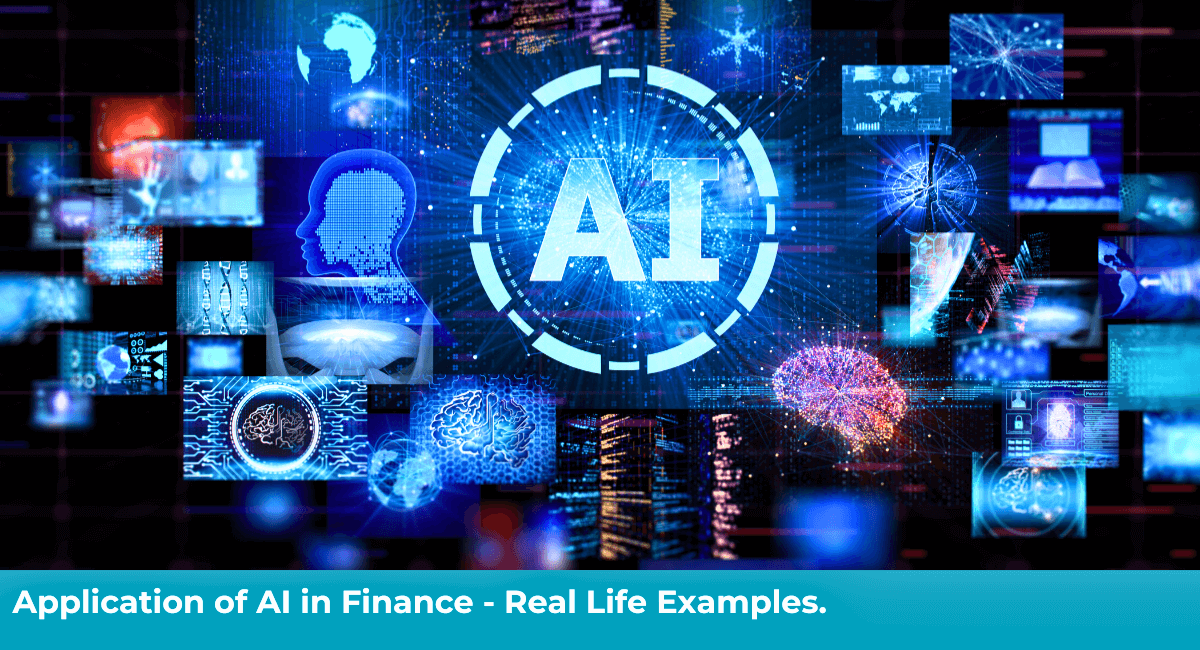
Algorithmic Trading
AI-driven algorithmic trading uses advanced machine learning models to trade faster and more precisely than humans. These models analyze vast amounts of market data in real time, look for patterns, and trade based on predefined criteria to make profits.
However, AI-driven trading systems must be monitored and updated to adapt to changing market conditions. Despite the advantages, these algorithms are still vulnerable to market volatility, flash crashes, and regulatory challenges, so they must be overseen.
- Example: Goldman Sachs, which uses AI-powered trading algorithms to make investment decisions. They have reduced their trading floor workforce and are using automated trading systems to execute complex financial strategies. An example uses AI-powered
Risk Management
AI is transforming risk management in the financial industry by making better decisions and preventing fraud. Banks and financial institutions use AI to assess credit risk, protect customer accounts, and anticipate financial threats.
Traditional credit scoring models are based on limited financial metrics, while AI models examine a broader range of data, including online behavior and transaction history. This results in more accurate creditworthiness assessments and fewer loan defaults and financial losses.
AI-powered machine learning models examine real-time transaction data to detect fraud. They identify unusual patterns and flag potential fraud. As they learn from new data, the models become more intelligent.
- Example: JPMorgan Chase uses AI-driven fraud detection systems to analyze millions of real-time transactions. Their AI models flag suspicious activity, helping to prevent unauthorized transactions and reduce financial risk. This proactive approach has increased security, regulatory compliance, and customer trust in digital banking.
Data Science and Analytics in Finance
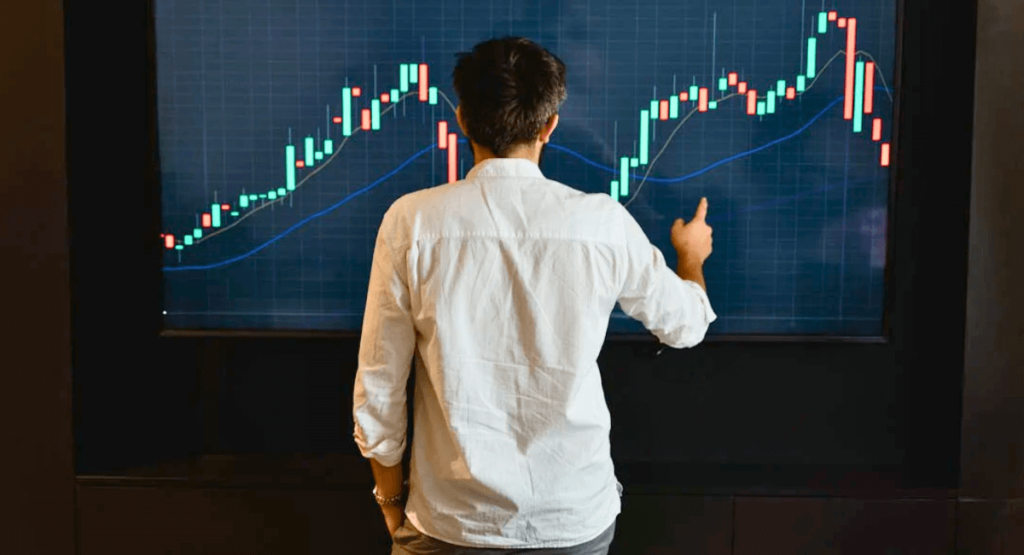
Data science and analytics are key to extracting insights from large amounts of data in finance. Advanced analytics allows financial institutions to gain business intelligence, make better decisions, and reduce risks.
One of the most significant benefits of data science in finance is identifying patterns and trends in financial data. Machine learning algorithms and predictive analytics can detect market movements, assess investment risks, and optimize portfolio strategies. Banks and financial institutions use these to analyze customer behavior, improve credit scoring, and enhance fraud detection.
Also, data-driven insights improve operational efficiency by automating risk assessment, compliance monitoring, and customer segmentation tasks. AI-powered analytics provides real-time forecasting and enables financial institutions to make proactive decisions.
- Example: JPMorgan Chase uses AI-powered analytics in its COiN platform to automate document review processes, reducing contract analysis time by 90%. This is how data science can streamline financial services.
Decision Support Systems in Finance
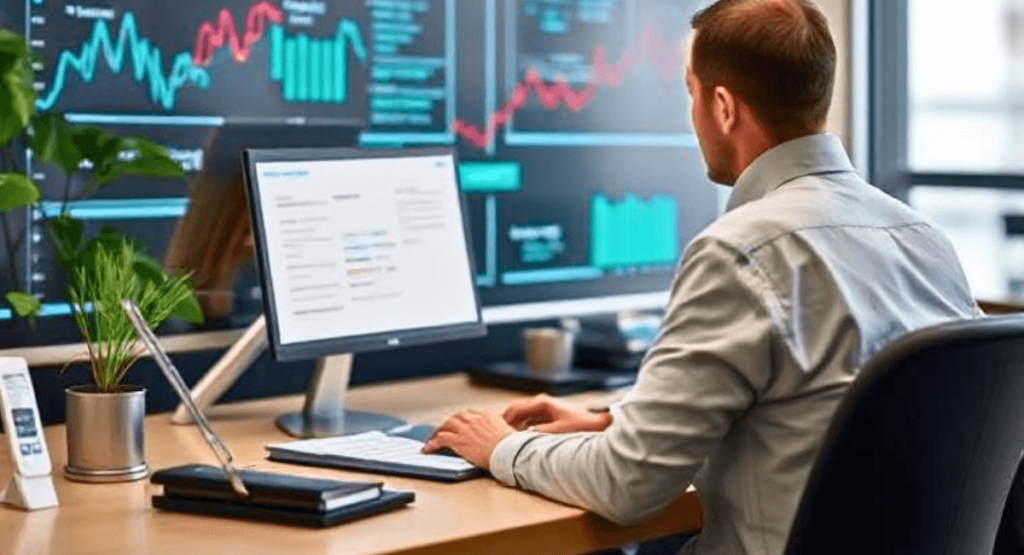
Decision Support Systems (DSS) help with financial decision-making by combining predictive analytics and data-driven models. Financial institutions can analyse complex data, assess risk, and optimise for better outcomes.
A DSS combines machine learning, artificial intelligence, and big data analytics to enhance strategic planning and risk management. In traditional banking, for example, DSS models predict loan defaults by analyzing credit history, transaction patterns, and economic trends. Banks can adjust their lending strategies, mitigate risk, and improve profitability.
Investment firms use DSS to analyse market trends and optimise portfolio management. These systems use real-time data tos help traders make informed investment decisions. DSS also helps with fraud detection by identifying suspicious activity and preventing financial crime.
- Example: Goldman Sachs utilizes AI-powered decision support systems (DSS) for risk management and trading strategies. The firm’s Marcus platform employs AI to evaluate credit risk and make real-time lending decisions. Goldman Sachs applies machine learning algorithms to enhance risk assessment accuracy and improve the customer experience. As markets grow more complex, DSS continues to develop, offering institutions intelligent insights and facilitating better decision-making in a rapidly changing economic landscape.
Cybersecurity and Fraud Detection in Finance
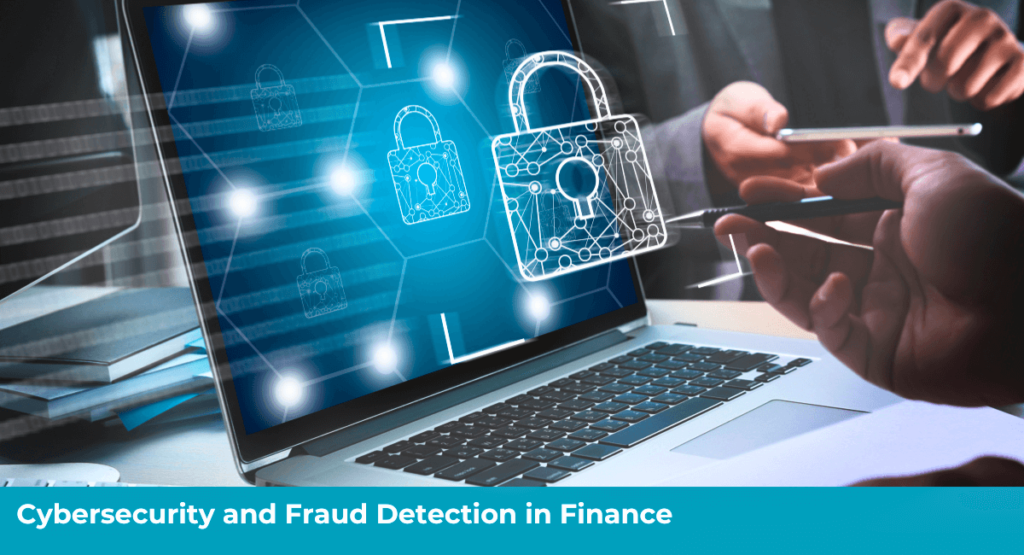
AI is key to securing online finance. Companies like Order.co and Ascent offer AI-based cybersecurity solutions to large financial institutions. AI can detect, prevent, and respond to cyber-attacks and threats.
- Example: Darktrace, an AI-driven cybersecurity company, uses machine learning to detect and neutralize cyber threats in real time. Its AI technology monitors financial transactions, detects suspicious activity, and mitigates risk before it becomes a breach. By continuously analyzing patterns and adapting to new threats, Darktrace helps financial institutions protect customer data and prevent fraudulent transactions.
AI-based cybersecurity solutions like this are essential for banks, payment platforms, and fintechs to protect from ever-evolving cyber risks. As threats get more sophisticated, AI will play an even more prominent role in securing financial systems and data integrity.
Blockchain and AI in Finance
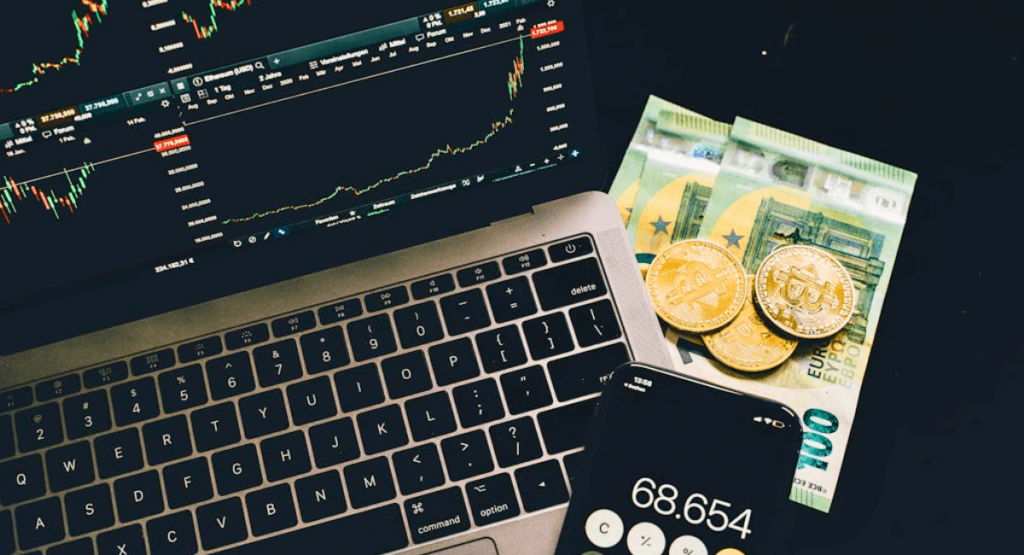
AI and blockchain are changing the face of finance by making it more secure, transparent, and efficient. AI can process and correlate data quickly, and when combined with blockchain’s decentralised and immutable ledger, this means safer and more reliable transactions. These technologies work together to detect fraud, automate processes, and optimize investment strategies.
- Example: Numerai, a hedge fund, uses AI and blockchain to crowdsource predictive models from data scientists worldwide. It uses encrypted financial data to train AI models and ensures security and privacy through blockchain. This approach improves trading strategies, reduces bias, and shows AI and blockchain in finance.
Challenges and Ethical Considerations in AI in Finance Professionals
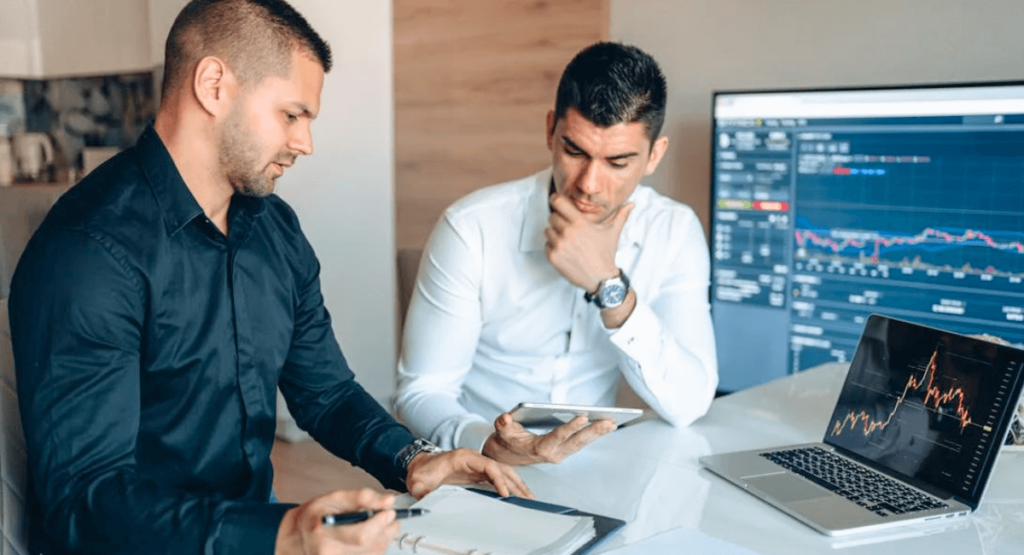
AI has changed the finance landscape, making it more efficient, accurate and effective in decision-making. But AI also brings big challenges and ethical issues along with it. The finance industry must address these to ensure fairness, security and compliance while harnessing AI.
Data Privacy and Security
AI needs lots of financial data to make predictions and automate decisions. But that’s where data privacy and security concerns come in. Sensitive financial information like personal banking details, transaction history and credit scores are at risk of unauthorized access or misuse.
Regulations like GDPR in the EU and CCPA in the US demand strict data protection. Financial institutions must ensure their AI-driven systems comply with these regulations, encrypt data, control access and have transparent data usage policies. Failure to comply can lead to legal consequences and loss of customer trust.
Bias and Fairness in AI Decision-Making
AI in finance is only as good as the data it’s trained on. The AI system will perpetuate or amplify discriminatory practices if the training data is biased. This is especially true for loan approvals, credit scoring and investment recommendations.
For example, AI models may unwittingly favor some demographic groups over others and unfairly lend. To mitigate bias, financial institutions need fair and unbiased data collection methods, audit AI models regularly and use explainable AI to ensure transparency in decision-making.
Lack of Transparency and Explainability
One of the biggest challenges in AI finance is the “black box” problem—the lack of understanding of how AI models make decisions. Many machine learning algorithms’ bottomless learning models are complex and not human-interpretable.
This lack of transparency raises ethical concerns as customers and regulators can’t know why a financial decision was made. To fix this, financial institutions use explainable AI (XAI) to give reasons for AI-driven decisions. Regulators also push for more transparency so AI models are accountable for their actions.
AI in Fraud Detection: Balancing Accuracy and Ethics
AI is crucial in fraud detection, identifying suspicious transactions, and reducing financial crimes. However, an overly aggressive AI system may generate false positives, flag legitimate transactions as fraudulent, and cause customers inconvenience.
For example, AI fraud detection in credit card transactions may block valid purchases based on unusual spending behavior, frustrating consumers. Striking a balance between fraud prevention and customer experience is essential. Financial institutions must continuously refine AI models, incorporating real-time feedback to minimize errors while maintaining robust security.
Ethical Considerations in AI in Finance
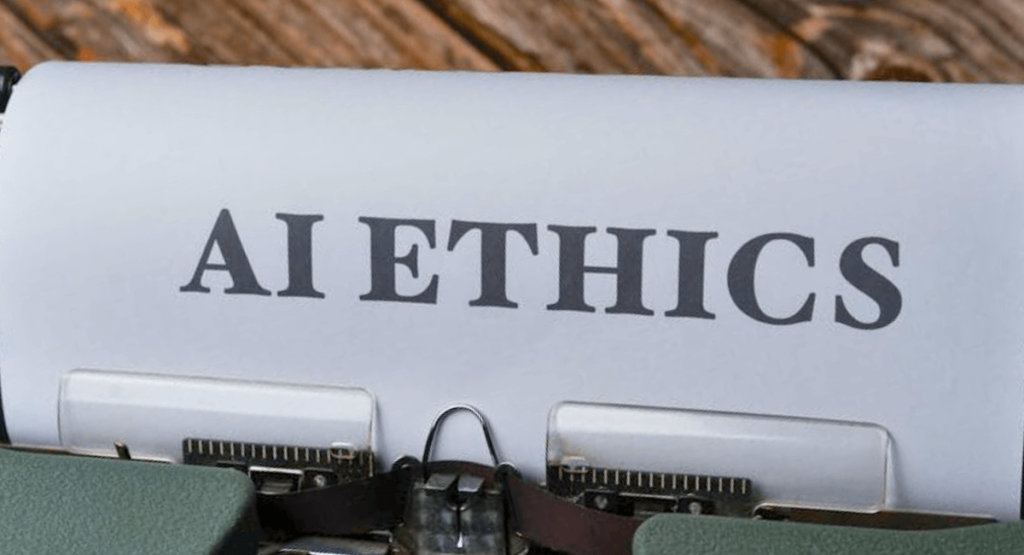
AI trading algorithms look at market trends and trade at lightning speed, giving institutions an edge. But HFT with AI has been criticized for causing market chaos and increasing the gap between institutions and retail investors.
Unethical AI trading strategies like market manipulation and price prediction biases can hurt the markets. Regulators need to set rules for ethical AI trading so AI can’t be misused for unfair advantages.
Regulatory Compliance and Governance
As finance gets more AI, regulatory complexity grows. Governments and institutions need to develop an AI governance framework to oversee ethical AI. Regulations should cover data privacy, bias mitigation, transparency, consumer protection, and innovation.
Many financial regulators like the SEC and FCA are coming out with guidelines for AI. Institutions need to get ahead of the curve by investing in ethical AI research, adopting responsible AI practices, and following evolving regulations.
Artificial Intelligence in the Financial Services Industry
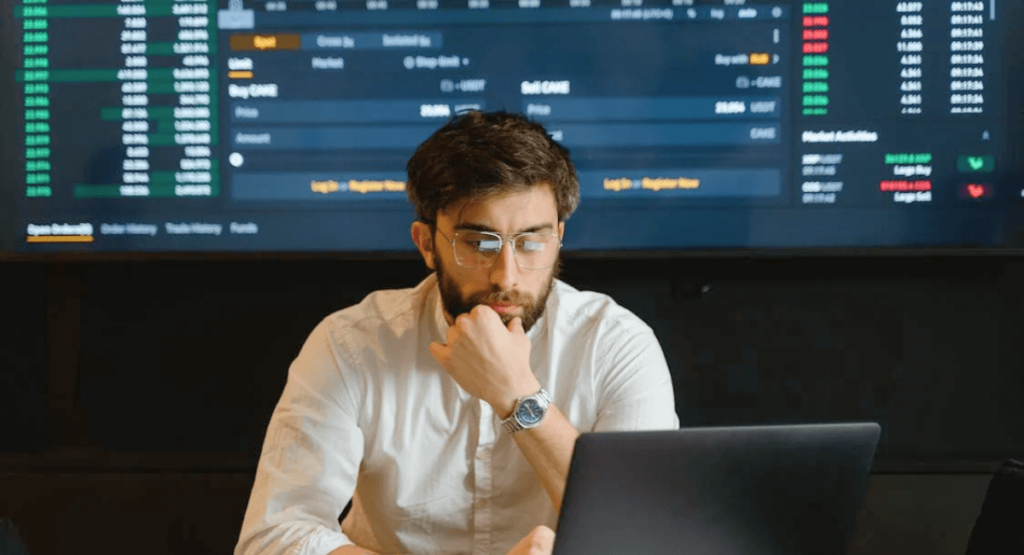
Artificial Intelligence (AI) will transform the financial markets through growth, efficiency, and better customer experiences. As financial organizations go digital, AI will automate processes, improve risk management, and deliver personalization at scale.
Customer Engagement
One of the most enormous benefits of AI in financial services is personalized customer engagement. Banks, insurance companies, and investment firms use AI to understand customer behavior and preferences and offer tailored financial solutions. AI-powered chatbots and virtual assistants provide real-time support, so customers get accurate and efficient service when needed.
AI personalization will go beyond simple recommendations. Financial institutions will use AI to create dynamic, relationship-based interactions and offer insights and guidance aligned with individual financial goals. This will build customer trust and loyalty.
AI in Risk and Fraud
AI can process and analyze vast amounts of financial data in real time, making it a powerful tool for risk assessment and fraud detection. Unlike traditional systems, machine learning models can better identify suspicious transactions, detect anomalies, and flag potential threats. AI will be key to financial security and reducing fraud as cyber threats evolve.
Moreover, AI will help institutions predict and manage risk by analyzing market trends, economic indicators, and historical data. This will allow institutions to make informed decisions and develop proactive risk management strategies.
Automating Financials
AI will automate financials, reducing manual work and increasing efficiency. It will also speed up and improve the accuracy of loan approvals, credit scoring, claims processing, and regulatory compliance. This automation will enhance operational efficiency and save costs for financial institutions, allowing them to allocate resources more effectively.
The Future of AI-Driven Financial Products
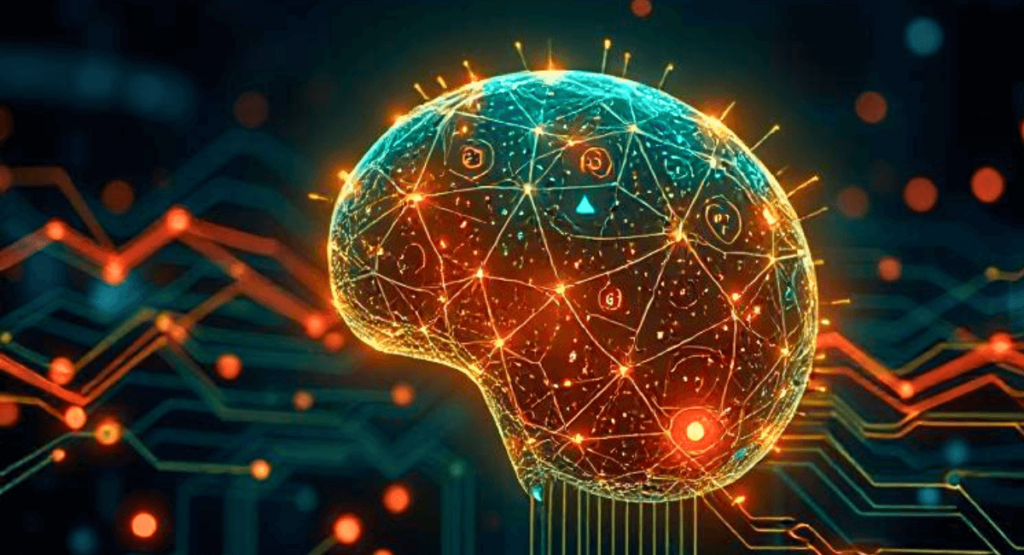
Financial institutions are exploring robo-advisors, AI-powered investment advice based on data analysis and market predictions. These digital advisors help individuals and businesses make informed decisions and reduce human bias.
Also, AI will expand concierge services and offer financial assistance when customers need it most. From AI wealth management to intelligent credit offerings, the future of financial services will be more intuitive, responsive, and customer-centric.
Conclusion
AI is changing the face of financial services by increasing customer engagement, risk management and automating core processes. It can analyze vast amounts of data so financial institutions can make better decisions, detect fraud, and offer personal financial solutions. As AI-driven automation reduces costs and increases efficiency, banks and financial institutions can focus on delivering seamless relationship-based services at scale.
However, ethical considerations like data privacy and bias must be managed to maintain trust and transparency. In the future, financial institutions need to balance innovation with responsible AI. By using AI ethically and effectively, the financial industry can create smarter, safer and customer-centric services, shaping the future of digital finance.
FAQs
How is AI used in Finance?
- AI is changing the financial services landscape by increasing customer engagement, improving risk management, and automating key processes.
How will AI change the Future of Finance?
- AI will enhance operational efficiency and save costs for financial institutions, allowing them to allocate resources more effectively.